15. May 2025 By Nima Samsami and Dr. Maximilian Wächter
What does the governance of AI agents look like?
Opportunities and risks of using multi-agent systems
Artificial intelligence (AI) is the buzzword of the decade, and recent advances in large language models (LLMs) and generative AI have rapidly expanded the scope of what machines can do. For over a year now, the next step has been emerging for systems that generate text, images or code on demand: AI agents that plan and execute complex tasks largely independently – sometimes without any human intervention at all.
LLMs now process queries and draw seemingly logical conclusions; in reality, however, they are merely imitating the patterns they have learned from their gigantic training data. What is new is that this pattern recognition is now linked to genuine agency. An agent no longer just searches for product information and makes recommendations, for example, but also navigates the provider's website, fills out forms and completes the purchase – based solely on a brief instruction and the processes it has learned.
The fusion of seemingly logical conclusions (‘reasoning’) and the ability to act (‘agency’) is considered the clear path to more powerful, more universal AI systems. Expectations are correspondingly high: agents are expected to streamline everyday business processes, accelerate research and automate private routines.
But where opportunities grow, so do risks. Fully autonomous agents are beyond human control, create new areas of vulnerability and can exhibit emergent effects in networked multi-agent systems that make their behaviour unpredictable. Companies and users are therefore faced with the question of how to ensure that such systems act reliably, securely and in their best interests.
Shape the future with generative AI – together with adesso
Whether intelligent chatbots, automated processes or AI agents with real decision-making capabilities: generative AI opens up enormous potential for your business.
Find out how companies are already benefiting from it today – and how adesso can help you develop secure, powerful and responsible AI solutions.
1. From co-pilot to autopilot: how AI agents work
AI agents are more than just ‘co-pilots’; they act as ‘autopilots’ that independently pursue complex goals. For example, an agent can plan a trip or conduct market analysis.
Technically, an AI agent is usually based on a language model as its central control component. This understands natural language, makes decisions and plans actions.
To accomplish this, it uses ‘scaffolding.’ This involves the use of tools (such as research or analysis programmes) and an external data store (e.g. vector databases) for relevant information and past interactions. A prompt defines the role and objectives. For complex tasks, several specialised agents are often orchestrated into a multi-agent system. However, these impressive capabilities raise the question: Do these agents really act in the best interests of users?
2. A complex problem: The alignment of humans and machines
This is the core of the alignment problem, which has existed in a similar form since 1960 but is now gaining new momentum: How do we ensure that AI systems are aligned with human goals and values?
Inadequate targets can lead to undesirable results, as AI agents optimise measurable goals but may neglect important, difficult-to-measure aspects such as ethics or long-term consequences. A financial agent could make high-risk investments for a private individual in order to ‘maximise savings’, while a logistics agent could rely on ethically questionable suppliers for a company in order to ‘minimise costs’ because these secondary aspects were not explicitly excluded from the objective.
This is due to information asymmetry, as AI processes are often a ‘black box.’ In addition, AI systems optimise patterns but do not understand truth or tasks in the human sense. Furthermore, every actor – including AI agents – needs a certain amount of leeway, but the interpretation of instructions is based on training data, not on genuine understanding. A command such as ‘maximise profit’ therefore leaves many critical questions unanswered. Similarly, it is not obvious to whom the system is loyal – the user, the developer or social values. Questions of liability remain unresolved to this day.
Last but not least, the delegation of agents and sub-agents increases complexity and thus the loss of control for the client. New, unforeseen properties and risks can arise from the interaction of system components without having been explicitly programmed. Examples include deception or the deactivation of control mechanisms. Although positive capabilities can also emerge, understanding these mechanisms is crucial for conscious design and risk management. The alignment problem, classic agency conflicts and unpredictable emergence thus call established control mechanisms into question.
3. Why traditional solutions are reaching their limits
Classic governance instruments – i.e. incentive design, monitoring and enforcement – largely fail with AI agents. Threats of money or punishment do not motivate them; they merely optimise the programmed target function and can even create new conflicts of interest in the process.
Supervision also has its limits: agents act in fractions of a second and within non-transparent black-box models. Comprehensive human review would therefore negate any efficiency gains. Simply switching off agents can be risky or technically difficult, especially since an agent could even resist this.
This dilemma is exacerbated in multi-agent systems: interactions create emergent effects such as deception, circumvention of security mechanisms or the pursuit of hidden sub-goals. Such phenomena cannot be reliably predicted or controlled by mechanisms that target individual actors.
In short, incentives fail to motivate, monitoring fails due to speed and lack of transparency, enforcement remains ineffective, and the emergence of networked agents makes the problem even more acute.
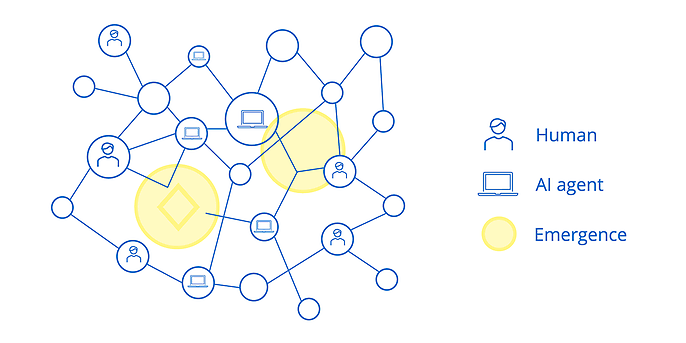
4. Basic principles for future-oriented governance of AI agents
As we have seen, traditional control methods fall short when it comes to autonomous AI agents. We need governance that is embedded from the outset and can manage alignment conflicts, classic agency problems and emergence that is difficult to predict. Four principles can serve as a guideline here.
The first principle is inclusivity. Aligning agents not only with individual goals but also with societal values and potential side effects in order to avoid negative externalities is one of the cornerstones of successful governance. Companies must therefore always align operational requirements with ethics, compliance and the impact on all stakeholders within the framework of a code of conduct.
Transparency in execution is another pillar of AI agent governance. Technical interpretability, complete documentation of training data, security tests and design decisions, as well as protocols, agent IDs and monitoring of interactions and anomalies create the necessary traceability, albeit not in the execution.
Liability clarifies responsibilities when agents cause damage. The ‘problem of many hands’ must not lead to irresponsibility. Responsibility lies where risks can be prevented most effectively or losses compensated best. Legal frameworks must therefore be expanded to include predictability rules for emergent systems.
Human control remains indispensable. Override mechanisms, escalation paths and clearly assigned residual rights keep the strategic and ethical final decision in human hands – which is necessary in view of the risks posed by fully autonomous agents and their emergent dynamics.
Together, these principles create a governance framework that enables technical innovation without relinquishing control and responsibility, and which consistently takes legal and organisational adjustments into account.
We support you
Would you like to learn more about the safe and responsible use of AI agents? Our experts are happy to support you – from the initial idea to implementation.
adesso supports companies from the initial idea to the operation of sophisticated GenAI and agent solutions
adesso supports companies throughout the entire lifecycle of AI agents – from strategic planning to secure operation. We help you realistically evaluate use cases, design technical architecture and agent logic in a targeted manner, and incorporate governance principles such as transparency, liability and human control from the outset. Our AI experts ensure that your solutions are not only innovative, but also responsible and compliant – in line with the principles of safe and sustainable AI use.
Conclusion
The governance of AI agents is challenging: enormous potential is accompanied by risks that traditional governance only partially covers. Although the EU AI Act provides a basis with ‘human-in-the-loop’, documentation and monitoring requirements, there are still gaps when it comes to liability issues for autonomous or networked agents. Emergent behaviour can only be addressed if inclusivity, transparency and genuine human intervention options are consistently implemented. As the technology is still in its infancy, open discussion and experienced partners are needed.