17. September 2021 By Ann-Kathrin Bendig and Jan Jungnitsch
From black box to white box
Explainable AI paves the way for using artificial intelligence in the insurance industry
Trust, protection, security.
In no other service industry is the customer’s need for protection, trust and security so strong as in the insurance industry. Due to the transfer of risk, the policy holder depends on the insurer’s promise of protection in the event of a claim and thus on the insurer’s performance to ensure they do not suffer acute financial difficulties. The refusal to pay a claim can mean economic ruin for many insured persons. Transparency regarding why certain decisions are made – either by humans or AI – is therefore a top priority in the insurance industry.
With eight regulatory authorities at national and EU level, insurers are among the most heavily regulated companies in Germany. It is clear that it is precisely these supervisory authorities that also influence progress and new business models. In connection with the use of artificial intelligence, the German Federal Financial Supervisory Authority (BaFin) makes it clear that black box applications are not accepted in models that are subject to supervision. The decision-making process concerning how AI arrives at a particular decision must be comprehensible and it should be possible to communicate it in a transparent manner for all parties. We need the black box to be converted into a white box. This is exactly where Explainable AI (XAI) enters the picture.
What is Explainable AI?
Explainable AI (XAI) is a methodical approach that facilitates the traceability of decisions required by customers and supervisory authorities. XAI explains the past, present and future actions of artificial intelligence. In short, XAI is about maximum transparency in the use of AI. The traceability of decisions gained by using XAI could significantly increase usability in the insurance industry and represent a milestone in the use of AI in the industry thanks to the fulfilment of legal requirements.
From black box to white box?
There are two methodological approaches within artificial intelligence. The classic approach is the black box, where training data is sent to a learning process. Although, as a user, I get a result from the AI, I do not usually receive an answer indicating why something was solved in the way it was. In the explainable approach, there is also a so-called abstraction layer. In other words, there is a model that works separately and extracts the results of the actual model on a different level. An explanatory interface ensures the user gets answers to the questions as to why and with what probability a particular decision was made. This means that I can understand and comprehend why the AI decides something in a certain way.
User groups are a new challenge
User groups pose a new challenge to XAI as they have varying requirements when it comes to explanations relating to the same model – depending on their role. Managers and executives expect answers at a strategic level of abstraction, and also measure the success rate (KPIs) of the model. The compliance of the model as well as its legal certainty are required by the regulatory entity. As an operational entity, the domain expert needs to trust the model in order to be able to carry out their day-to-day business and derives their own actions from it. In addition, there are users, who, in a strict sense, have the role of policyholder, and have to understand the decisions made by AI on a completely different level of abstraction, as they are lay persons and have no knowledge of either the model or learning procedures. The basis of decisions must be explained differently to these users. Other issues come into play here. For example, a decision should be fair and trustworthy and must also take into account the privacy of the user. Finally, there are the actual technicians such as data scientists, product owners and software engineers who have extensive technical expertise and control the transformation process.
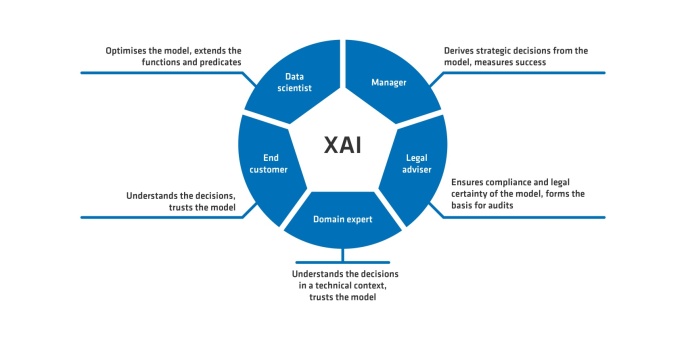
With XAI, a different, new approach is ultimately required, as the level of abstraction and explanation needs to be addressed in a deeper and more comprehensive way. This also increases the qualifications of the data scientists in terms of their professionalism and expertise, as the attention paid to the abstraction level (GUI) for the user becomes more important.
These XAI methods are undoubtedly a prerequisite for artificial intelligence to be used by insurers, as the demand for transparency and the basic insurance-specific idea of trust, protection and security can only be fulfilled through this explanatory work.
You would like to learn more about exciting topics from the adesso world? Then take a look at our previously published blog posts.